16 min read • Organization & transformation, Technology & innovation management
The people-centric lab of the future
AI-supported innovation to meet tomorrow’s needs

As the world grapples with global mega-challenges, innovation[1] has never been more necessary. We need breakthrough innovation to solve pressing issues around energy, the environment, and social and health challenges. At the same time, and equally importantly, companies have to deal with a business world typified by increased complexity and accelerated time frames. Together these mean innovation must deliver more breakthroughs, rather than only incremental improvements, with more flexibility and responsiveness and at greater speed.
However, these breakthrough innovations require new approaches that go beyond traditional ways of working. Our research in the Global Innovation Excellence Benchmark[2] shows that more than 80% of companies are unsatisfied with their return on breakthrough innovation investments. Many CEOs and CTOs that we speak to say their innovation pipeline isn’t nearly enough to support their company’s growth ambitions.
In “The Laboratory of the Future,”[3] our 2020 Prism article, we argued for the need to take a new, people-centric approach to digitalized R&D (by “lab,” we mean the entire “delivery engine” of R&D). We made the point that to meet the challenges of increasing convergence, as well as the need for more and more rapid breakthroughs, the lab had to be designed around people, rather than technologies or systems.
Since then, the sudden acceleration of artificial intelligence (AI) has started to transform how we do business, with innovation and R&D being no exception. Hardly a week goes by without the announcement of yet another fascinating AI use case — one example is “Coscientist,” an AI-driven approach that combines different GPT-4 models and is capable of designing, planning, and executing experiments in the context of chemical research[4]. A second, equally fascinating example is “FunSearch,” an AI approach that combines a large language model (LLM) with an evaluator module in the search for novel solutions to open problems in the world of mathematics[5].
While these two examples are fairly specialist and mostly driven by academic research groups, the broader potential of AI to transform innovation and R&D is huge. We can already see the effect that generative AI is having on access to and democratization of knowledge. AI is rapidly becoming a general-purpose technology with enormous potential impact, akin to the advent of electricity and the internet in the 19th and 20th centuries, respectively, when entire business sectors were transformed forever and fortunes were made by early vendors and adopters.
However, it will take years or even decades before companies and industry standards will have converged toward any single “best practice” in its use. Such future standards will also need to address concerns such as (cyber)security, accuracy, and “explainability.” These are not the main topic of this article, but certainly important to consider[6].
In the meantime, the companies that are most adept at identifying, accessing, and broadly deploying leading AI technology and use cases within R&D will see enormous benefits. This article considers the “big ticket” potential of AI to innovate how innovation is done. In particular, we will explore the importance of putting people at the heart of AI-augmented innovation, supporting them with the structures, organizational models, and optimal conditions to enable them to become perfect innovators, uniquely placed to bring the breakthrough innovations that the world is waiting for.
How AI Can Help Innovate how Innovation Is Done
The list of challenges to creating a steady stream of breakthrough innovations is long and potentially dispiriting. Success rates are low, with lead times often excruciatingly long and the ability of companies to realize the full potential of successful technology breakthroughs low.
So how could AI change this picture? Examples of successful AI applications in R&D are already plentiful. Uses range from ideation and literature-based discovery; through novel concept creation and in silico design and experimentation, rapid candidate screening in drug discovery, trend analysis, and pattern recognition; to prototype development, design optimization, and testing support. Multiple LLMs can be bundled and/or combined with other systems and APIs to support almost any task a human researcher would undertake. AI use is greatly bolstered by the fact that it is able to “read” almost any type of unstructured information in a multimodal fashion, such as complex tables, charts, and drawings.
Progress will not stop there. Based on generative AI’s seven archetypes of human intelligence[7], we expect innovation teams to primarily benefit from using AI in these typical roles:
-
Scribe: generating content across all media, based on specific prompts
-
Librarian: answering questions, lookup, and search
-
Analyst: summarizing data series and underpinning pattern recognition and extrapolation
-
Engineer: aiding task definition, analysis, and optimization for problem solving
-
Scientist: causal inference of general laws based on empirical observation and counterfactual reasoning
-
Craftsman: directing and manipulating physical tools and objects in uncontrolled environments
-
Artist: aiding ideation and design, bringing together diverse influences and perspectives to help innovators create original ideas and concepts
While it is easy to see how these AI applications could increase innovation productivity and efficiency, if they are simply plugged into a “traditional” lab setting, they won’t in themselves bring about the transformation needed to meet today’s and tomorrow’s needs. For the foreseeable future at least, AI will be a copilot or assistant to the human beings running the lab. To get the most out of AI, innovation and R&D organizations need to focus on people.
Building the Laboratory of the Future — People Before Technology
The lab of the future is the target destination for any large organization aspiring to break out of the traditional R&D mold. At its core, it aims to make people into “perfect innovators” to realize strategic objectives, akin to automatic driving aids aiming to make people into “perfect drivers.” This is about much more than offering technology and hoping for the best. Instead, it means re-engineering a fully people-centric lab approach, enabled by AI and other digital tools, based around three key components: democratization, collaboration, and ambidexterity (see Figure 1).
In essence, the concept is straightforward:
-
Democratization involves making AI available, customized for everyone and adopted as a natural way of working, closing the gap between AI users and AI providers.
-
Collaboration involves using AI and other digital tools to help supercharge the sharing of data and knowledge, forming a virtuous circle unconstrained by the limitations of current data management.
-
Ambidexterity involves setting the organizational context to facilitate and encourage AI-supported breakthrough innovation and exploration, at the same time as maintaining R&D efficiency and productivity.
While these three components can be considered separately, they are connected and mutually reinforcing: democratization requires pervasive sharing of data and knowledge, which, in turn, requires organizational ambidexterity, and so on.
Democratization
In 2023, access to and interaction with powerful AI systems such as LLMs became democratized. For example, advances in reinforcement learning through human feedback (RLHF) have enabled easy conversational access to these very powerful models for everyone. AI usage is no longer exclusive to very large companies, but has become available to the wider world. The fact that Open AI’s ChatGPT model managed to attract more than 175 million users in one year is a clear testament to this.
When considering AI’s potential through a people-centric lens, it helps to start by defining what makes people, and innovators in particular, truly good at their job. The T-shape (see Figure 2) has often been used as a model to represent a person’s specific areas of expertise (vertical), as well as more interdisciplinary collaboration skills (horizontal). The same model can be applied to the AI models and bots required to support people in their daily jobs (as highlighted in a previous Prism article[8]).
AI, when integrated into researchers’ natural ways of working, can unlock the promise of complete knowledge integration, in combination with step changes in the pace and reach of knowledge expansion. This is key to developing broader technology portfolios, greater technology advances, more breakthrough and business model innovations, shorter time to market, and the ability to address larger markets.
AI can be applied to enhance knowledge integration in several ways, for example:
-
Accelerating solution engineering: Thanks to AI, the ability to access and synthesize sources for insights becomes potentially limitless. Transversal solution design skills determine the ultimate impact of solution engineering. For example, General Motors reports that it is combining generative AI, working mainly in the craftsman role, with additive manufacturing to drastically extend the design space to be explored for novel parts and components.
-
Enabling co-creation: AI can fulfill the role of analyst and engineer in co-creation with humans, especially in systems engineering, which requires the involvement of multiple stakeholders, many of which are non-technical, such as in model-based systems engineering. Smart cities are one example of this type of application.
-
Blending of formal and informal knowledge streams: AI can help spark ideation and increase problem solving by integrating formal and informal knowledge streams. While formal knowledge is already encoded in data (being structured or unstructured in nature), informal/tacit knowledge can be inferred through interaction patterns. For example, this could be facilitated through transcription of brainstorming sessions or using a generative AI model as a “sparring partner” in ideation processes. The greater knowledge transparency provided through AI helps with innovation decision making: for example, direction setting (where to look), priority setting (where to go deeper), and opportunity validation (where to develop).
Turning to knowledge expansion, AI can also help in multiple ways, for example:
-
Hyperspecialized learning: Creating specialized and highly personalized AI models (working as scribe and librarian) will accelerate learning on subject matter expertise, increasing the scale and spread of critical knowledge pockets. The almost personal nature of these LLMs will most likely mean that they will be able to run on end user devices and be readily available whenever a user wishes to interact with them. A recent paper published by researchers from Apple[9] highlights a novel approach to running LLMs for inference on low-powered edge devices. This will most likely mean we will have a personal GPT available on our iPhones in the near future.
-
Augmentation of project teams: AI’s predictive capabilities greatly improve the effectiveness and speed of experimentation. Generative AI routinely fulfills the role of engineer and scientist, augmenting increasingly complex human tasks. This evolution toward hybrid ecosystems (of subject matter experts and bots) will require dedicated leadership, as well as continuous development and maintenance, to remain effective. The “Coscientist” approach recently published by researchers at Carnegie Mellon University is a great example of this. They have combined several LLMs for problem solving, code generation, and experimental design, with the ultimate goal of accelerating R&D in chemical research.
-
Fluidity of problem solving approaches: Librarian-like AI will vastly increase the visibility of alternative approaches and technologies to overcome technical hurdles, for instance, by finding solutions to similar problems in unrelated industries. This will have significant implications for project team composition and mentality. The application of generative AI approaches to well-established problem solving frameworks, such as TRIZ, could create significant productivity gains in this domain.
Across both dimensions, the design and interface of AI tools is vital if they are to achieve widespread user adoption, and thus deliver benefits. They have to be integrated within existing ways of working that staff are comfortable with and augment their capabilities. For instance, one of our clients (a large chemical player) has developed a transversal LLM team to support both “horizontal” and “vertical” initiatives across its vast R&D community.
Collaboration
The limitations of current collaborative data sharing and knowledge management systems have been amply described. Put simply, critical knowledge and data are often not accessed and used because they:
-
Are not properly codified/tagged
-
Sit in inaccessible locations
-
Exist in different file formats and/or different languages
-
Are “tacit” (i.e., part of people’s professional experience)
-
Are spread across multiple different ecosystem players
-
Are not being sought or shared by individuals for a variety of reasons
These obstacles are significant for deploying AI to help create perfect innovators because AI needs data by the truckload and people need new knowledge and insights to make new discoveries. Fortunately, AI and other digital tools are also part of the solution, for example:
-
Advanced knowledge search: LLMs can be combined with knowledge graphs and vector databases to create new solutions that combine natural language processing with content and contextual search. ADL has recently deployed this type of tool for its own internal use.
-
Intelligent triggers: AI tools can be applied to provide prompts to initiate the transfer of information or catalyze learning activities. Examples include predicting users’ future needs and prompting action, providing tailored knowledge debrief protocols at key milestones, and automating translation and tagging to generate quick reference lists for specific topics.
-
Knowledge management aids: AI can support documentation, search, transfer, and learning. For example, generative AI can suggest non-obvious and/or lateral information in searches and generate new content from unstructured data (such as text/video/audio) for knowledge capture. Graph data technology makes it simpler to identify previously hidden relationships within vast amounts of textual data within repositories.
-
User experience enhancement: New tools can be deployed to make interfaces easier to use than not to use. For example, adoption engineering can be employed to make sure knowledge management and learning solutions “go viral” and deliver behavioral change with seekers, while gamification encourages owners to share information. Within repositories, powerful data visualization interfaces make understanding and prioritizing information easier.
Ambidexterity
While simply applying AI technology and tools to an existing lab structure has already had benefits, it is unlikely to result in any major breakthroughs (or even smaller ones) unless necessary changes are also made to the wider organizational context and operating model. After all, why would AI-enabled breakthroughs sail by well-known obstacles that plague other types of breakthrough innovations, such as inept decision making and misaligned incentives?
The lab of the future needs to embrace ambidexterity, balancing optimal exploitation of today’s innovation space and exploration of tomorrow’s growth domains — indeed, this why the T-profile of knowledge integration and knowledge expansion is essential.
R&D organizations that are geared toward exploitation tend to apply standardized formal structures and processes, supported by a performance-driven culture with KPIs focused on efficiency, cost reduction, scale, and productivity.
Those that are focused on exploration tend to have more lateral and fluid structures to allow greater responsiveness (for example, based on communities of expertise or guilds). Processes follow agile thinking and focus on iteration, rapid validation of ideas/sparks, and more freedom to follow “big bet” projects. Steering and incentives are more rooted in purpose and direction setting than individual project control.
Most R&D organizations, in practice, lean toward one of these archetypes or the other. ADL’s “Ambidextrous Organization Assessment database” finds that only 15% of companies are fully ambidextrous, with the majority (55%) optimized for exploitation (scale and productivity). A large majority of industrial R&D organizations still need to achieve ambidexterity, yet without it, the full potential of AI is unlikely to be realized.
So, what are the key features of ambidexterity in practice? Apple provides some useful pointers.
Lessons learned from Apple in ambidexterity
Apple is a longstanding innovation leader. We recently benchmarked Apple’s R&D function through interviews and desk analysis, confirming the importance of ambidexterity to its success. Notable features of its practices include the following:
-
Ambidexterity in decision making and performance management: Decision making depends on the type of project: more belief-based for design initiatives versus hard data to drive technical hardware specs. The steering of individual projects is much more closely linked to the ambitions and expectations of leaders (see next point) than dedicated KPIs, with team reputation seen as a more effective “control mechanism” than fixed indicators. R&D employee incentives are usually based on overall company performance rather than individual projects/products.
-
Ambidexterity in people management: Leaders are required to balance deep expertise and immersion in details with willingness to collaborate, with staff continuously assessed on these criteria. Apple follows the “experts lead experts” rule, requiring leaders to constantly learn in new areas as they become relevant to their area of responsibility. Clear distinctions have been made between topics to own, delegate, learn, and teach.
-
Ambidexterity in structure: Despite its size, Apple is purposely following a functional, rather than project-based, organizational model. In combination with other practices, it uses an expertise-driven approach and reduces the number of executives involved in decision making. While it still adopts project-driven ways of working, rather than fully formalizing this through organizational structures, it places much emphasis on a few selected project leadership roles, which are taken very seriously.
Comparing Apple R&D to a typical industrial company shows major differences in the ambidexterity balance (see Figure 3).
Two findings stick out from the comparison. First, whereas Apple R&D has embedded both “explore” and “exploit” in all organizational dimensions, industrial companies tend to optimize for “exploit” following predictable and well-controlled practices. Second, many industrial R&D organizations have dedicated units, and sometimes locations, designed for breakthrough innovation (“explore”), but this does not seem to be Apple’s formula for breakthrough success, which instead resides more in its whole approach to steering and transformation and managing people and culture.
Finally, Apple also exhibits the other key characteristics of the people-centric lab of the future, which we have already discussed. AI democratization and leadership are fully embedded within its functional organization as key guiding principles:
-
Democratization: A distributed (democratized) approach to AI classifies it as a crucial skill and requires the broader leadership to reach critical skill levels. It has no separation between “innovating with AI” (for example, increasing R&D productivity) and “innovations with AI” (such as improving the Siri functionality of iPhones).
-
Collaboration: Apple embraces its knowledge ecosystem, with high permeability in terms of AI experts moving in and out of the company routinely. It relies heavily on a managed ecosystem of high tech and software companies, academia, and startups.
Insights for the Executive
The scope and potential of the AI-driven, people-centric lab of the future is enormous. Resources are always limited and often spread thinly, which makes prioritizing (what is most important) and phasing (what do we do first) according to what matters most for the company’s strategic objectives crucial.
The guiding principle is “build for advantage, buy for parity”: focus efforts on must-win innovation battles based on superior technical capabilities, while adopting proven best practices and commercially available solutions for less important topics. For example, a pharmaceutical company may decide to deploy AI mainly to supercharge its core R&D activity of new drug discovery, while a food ingredient company might focus on AI-enhanced digital services around personalized nutrition and formulation. The principle is the same, but the focus is different.
These strategic innovation priorities can then be mapped to the T-shape model presented in this article: what are the implications to AI-driven knowledge integration and expansion? Do not get bogged down in technologies at this stage, but instead concentrate on what would need to happen along these two dimensions to make your people into “perfect innovators.”
This will give you the solution promises of AI that are specific to your company, which, in turn, will define the blueprint for your people-centric lab of the future. Focus on developing the three key components:
1. Democratization
-
Identify and prioritize AI use cases and adoption, integrated across the R&D and innovation cycle, with defined AI skill-level targets and competences.
-
Focus on end-to-end processes so technical experts can seamlessly adopt AI into their working routines.
-
Ensure no separation between “innovating with AI” and “innovations with AI.”
2. Collaboration
-
Develop and build out future requirements in terms of data architecture and engineering, as well as user experience.
-
Strengthen and diversify the wider innovation ecosystem and increase permeability between roles, teams, and the company at large.
-
Redefine the skills and expertise that are needed and use this to drive hiring, training, and team composition.
3. Ambidexterity
-
Strengthen the role and caliber of project leadership and solution engineering and increase the iteration steps in end-to-end innovation with more frequent optimization.
-
Focus on optimized decision making — reduce hierarchies and redesign governance to differentiate between types of decisions.
-
Foster an innovation culture that is familiar and comfortable with uncertainty and ambiguity — essential for being able to experiment and learn along the AI adoption journey.
AI is often portrayed in the popular media as a threat to people, threatening to take over their jobs and make them surplus to requirements. While the far future is impossible to predict, in the coming years at least, AI is paradoxically one of the most powerful tools we have to put people back at the center of industrial enterprise, where they belong.
Notes
[1] In this article we will use the words “innovation” and “R&D” more or less interchangeably. With the former we imply a broader concept of which R&D can be considered an integral part.
[2] 9th Arthur D. Little Global Innovation Excellence Benchmark, June 2023 https://www.adlittle.com/uk-en/insights/report/good-great-enhancing-innovation-performance-through-effective-management-processes
[3] “The Laboratory of the Future,” Prism S1 2020 https://www.adlittle.com/uk-en/insights/prism/laboratory-future
[4] https://www.nature.com/articles/s41586-023-06792-0
[5] https://www.nature.com/articles/s41586-023-06924-6
[6] See “Be Careful Out There — Understanding the Risks of Deploying Artificial Intelligence” elsewhere in this issue.
[7] “Generative Artificial Intelligence: Toward a New Civilization?” Blue Shift Report, October 2023 https://www.adlittle.com/en/insights/report/generative-artificial-intelligence-toward-new-civilization
[8] “Taking Control of AI,” Prism S2 2023 https://www.adlittle.com/sites/default/files/prism/ADL_PRISM_S2_2023_Taking%20control%20of%20AI.pdf
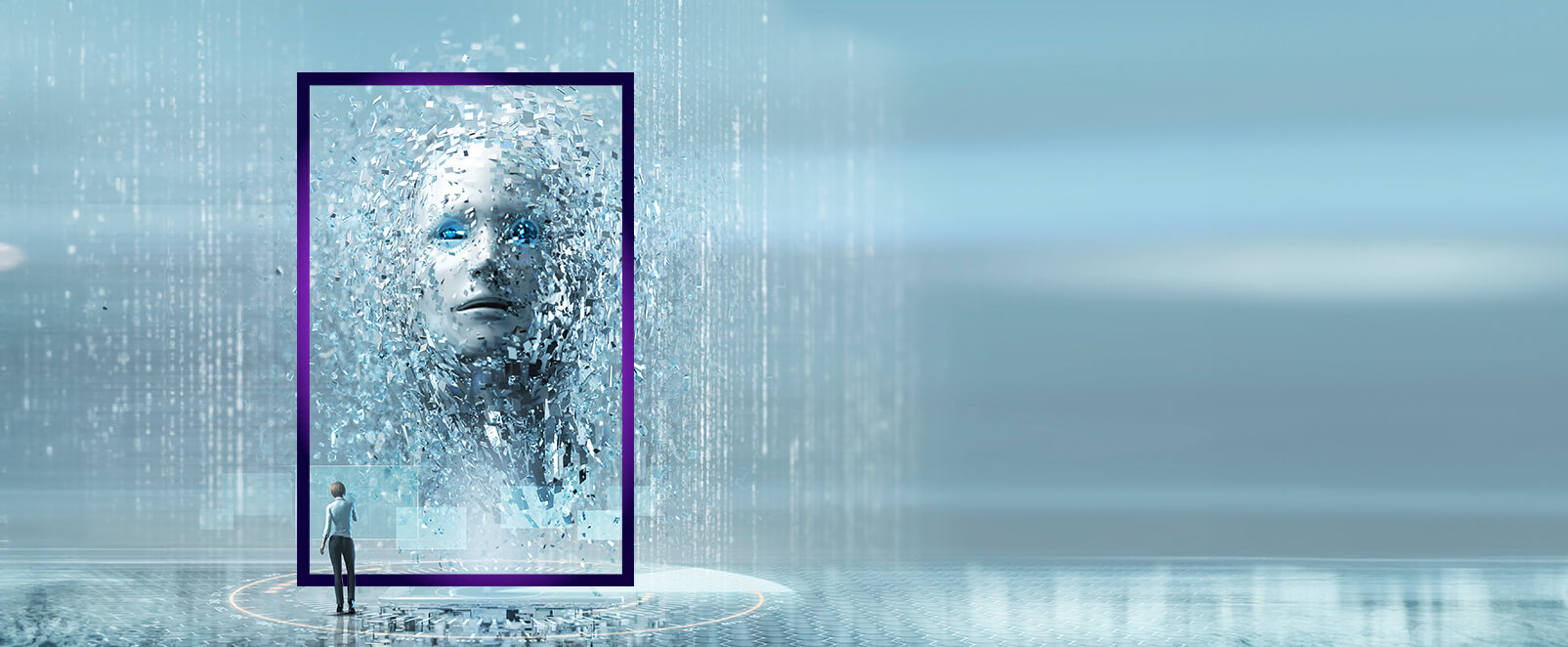
16 min read • Organization & transformation, Technology & innovation management
The people-centric lab of the future
AI-supported innovation to meet tomorrow’s needs

DATE

As the world grapples with global mega-challenges, innovation[1] has never been more necessary. We need breakthrough innovation to solve pressing issues around energy, the environment, and social and health challenges. At the same time, and equally importantly, companies have to deal with a business world typified by increased complexity and accelerated time frames. Together these mean innovation must deliver more breakthroughs, rather than only incremental improvements, with more flexibility and responsiveness and at greater speed.
However, these breakthrough innovations require new approaches that go beyond traditional ways of working. Our research in the Global Innovation Excellence Benchmark[2] shows that more than 80% of companies are unsatisfied with their return on breakthrough innovation investments. Many CEOs and CTOs that we speak to say their innovation pipeline isn’t nearly enough to support their company’s growth ambitions.
In “The Laboratory of the Future,”[3] our 2020 Prism article, we argued for the need to take a new, people-centric approach to digitalized R&D (by “lab,” we mean the entire “delivery engine” of R&D). We made the point that to meet the challenges of increasing convergence, as well as the need for more and more rapid breakthroughs, the lab had to be designed around people, rather than technologies or systems.
Since then, the sudden acceleration of artificial intelligence (AI) has started to transform how we do business, with innovation and R&D being no exception. Hardly a week goes by without the announcement of yet another fascinating AI use case — one example is “Coscientist,” an AI-driven approach that combines different GPT-4 models and is capable of designing, planning, and executing experiments in the context of chemical research[4]. A second, equally fascinating example is “FunSearch,” an AI approach that combines a large language model (LLM) with an evaluator module in the search for novel solutions to open problems in the world of mathematics[5].
While these two examples are fairly specialist and mostly driven by academic research groups, the broader potential of AI to transform innovation and R&D is huge. We can already see the effect that generative AI is having on access to and democratization of knowledge. AI is rapidly becoming a general-purpose technology with enormous potential impact, akin to the advent of electricity and the internet in the 19th and 20th centuries, respectively, when entire business sectors were transformed forever and fortunes were made by early vendors and adopters.
However, it will take years or even decades before companies and industry standards will have converged toward any single “best practice” in its use. Such future standards will also need to address concerns such as (cyber)security, accuracy, and “explainability.” These are not the main topic of this article, but certainly important to consider[6].
In the meantime, the companies that are most adept at identifying, accessing, and broadly deploying leading AI technology and use cases within R&D will see enormous benefits. This article considers the “big ticket” potential of AI to innovate how innovation is done. In particular, we will explore the importance of putting people at the heart of AI-augmented innovation, supporting them with the structures, organizational models, and optimal conditions to enable them to become perfect innovators, uniquely placed to bring the breakthrough innovations that the world is waiting for.
How AI Can Help Innovate how Innovation Is Done
The list of challenges to creating a steady stream of breakthrough innovations is long and potentially dispiriting. Success rates are low, with lead times often excruciatingly long and the ability of companies to realize the full potential of successful technology breakthroughs low.
So how could AI change this picture? Examples of successful AI applications in R&D are already plentiful. Uses range from ideation and literature-based discovery; through novel concept creation and in silico design and experimentation, rapid candidate screening in drug discovery, trend analysis, and pattern recognition; to prototype development, design optimization, and testing support. Multiple LLMs can be bundled and/or combined with other systems and APIs to support almost any task a human researcher would undertake. AI use is greatly bolstered by the fact that it is able to “read” almost any type of unstructured information in a multimodal fashion, such as complex tables, charts, and drawings.
Progress will not stop there. Based on generative AI’s seven archetypes of human intelligence[7], we expect innovation teams to primarily benefit from using AI in these typical roles:
-
Scribe: generating content across all media, based on specific prompts
-
Librarian: answering questions, lookup, and search
-
Analyst: summarizing data series and underpinning pattern recognition and extrapolation
-
Engineer: aiding task definition, analysis, and optimization for problem solving
-
Scientist: causal inference of general laws based on empirical observation and counterfactual reasoning
-
Craftsman: directing and manipulating physical tools and objects in uncontrolled environments
-
Artist: aiding ideation and design, bringing together diverse influences and perspectives to help innovators create original ideas and concepts
While it is easy to see how these AI applications could increase innovation productivity and efficiency, if they are simply plugged into a “traditional” lab setting, they won’t in themselves bring about the transformation needed to meet today’s and tomorrow’s needs. For the foreseeable future at least, AI will be a copilot or assistant to the human beings running the lab. To get the most out of AI, innovation and R&D organizations need to focus on people.
Building the Laboratory of the Future — People Before Technology
The lab of the future is the target destination for any large organization aspiring to break out of the traditional R&D mold. At its core, it aims to make people into “perfect innovators” to realize strategic objectives, akin to automatic driving aids aiming to make people into “perfect drivers.” This is about much more than offering technology and hoping for the best. Instead, it means re-engineering a fully people-centric lab approach, enabled by AI and other digital tools, based around three key components: democratization, collaboration, and ambidexterity (see Figure 1).
In essence, the concept is straightforward:
-
Democratization involves making AI available, customized for everyone and adopted as a natural way of working, closing the gap between AI users and AI providers.
-
Collaboration involves using AI and other digital tools to help supercharge the sharing of data and knowledge, forming a virtuous circle unconstrained by the limitations of current data management.
-
Ambidexterity involves setting the organizational context to facilitate and encourage AI-supported breakthrough innovation and exploration, at the same time as maintaining R&D efficiency and productivity.
While these three components can be considered separately, they are connected and mutually reinforcing: democratization requires pervasive sharing of data and knowledge, which, in turn, requires organizational ambidexterity, and so on.
Democratization
In 2023, access to and interaction with powerful AI systems such as LLMs became democratized. For example, advances in reinforcement learning through human feedback (RLHF) have enabled easy conversational access to these very powerful models for everyone. AI usage is no longer exclusive to very large companies, but has become available to the wider world. The fact that Open AI’s ChatGPT model managed to attract more than 175 million users in one year is a clear testament to this.
When considering AI’s potential through a people-centric lens, it helps to start by defining what makes people, and innovators in particular, truly good at their job. The T-shape (see Figure 2) has often been used as a model to represent a person’s specific areas of expertise (vertical), as well as more interdisciplinary collaboration skills (horizontal). The same model can be applied to the AI models and bots required to support people in their daily jobs (as highlighted in a previous Prism article[8]).
AI, when integrated into researchers’ natural ways of working, can unlock the promise of complete knowledge integration, in combination with step changes in the pace and reach of knowledge expansion. This is key to developing broader technology portfolios, greater technology advances, more breakthrough and business model innovations, shorter time to market, and the ability to address larger markets.
AI can be applied to enhance knowledge integration in several ways, for example:
-
Accelerating solution engineering: Thanks to AI, the ability to access and synthesize sources for insights becomes potentially limitless. Transversal solution design skills determine the ultimate impact of solution engineering. For example, General Motors reports that it is combining generative AI, working mainly in the craftsman role, with additive manufacturing to drastically extend the design space to be explored for novel parts and components.
-
Enabling co-creation: AI can fulfill the role of analyst and engineer in co-creation with humans, especially in systems engineering, which requires the involvement of multiple stakeholders, many of which are non-technical, such as in model-based systems engineering. Smart cities are one example of this type of application.
-
Blending of formal and informal knowledge streams: AI can help spark ideation and increase problem solving by integrating formal and informal knowledge streams. While formal knowledge is already encoded in data (being structured or unstructured in nature), informal/tacit knowledge can be inferred through interaction patterns. For example, this could be facilitated through transcription of brainstorming sessions or using a generative AI model as a “sparring partner” in ideation processes. The greater knowledge transparency provided through AI helps with innovation decision making: for example, direction setting (where to look), priority setting (where to go deeper), and opportunity validation (where to develop).
Turning to knowledge expansion, AI can also help in multiple ways, for example:
-
Hyperspecialized learning: Creating specialized and highly personalized AI models (working as scribe and librarian) will accelerate learning on subject matter expertise, increasing the scale and spread of critical knowledge pockets. The almost personal nature of these LLMs will most likely mean that they will be able to run on end user devices and be readily available whenever a user wishes to interact with them. A recent paper published by researchers from Apple[9] highlights a novel approach to running LLMs for inference on low-powered edge devices. This will most likely mean we will have a personal GPT available on our iPhones in the near future.
-
Augmentation of project teams: AI’s predictive capabilities greatly improve the effectiveness and speed of experimentation. Generative AI routinely fulfills the role of engineer and scientist, augmenting increasingly complex human tasks. This evolution toward hybrid ecosystems (of subject matter experts and bots) will require dedicated leadership, as well as continuous development and maintenance, to remain effective. The “Coscientist” approach recently published by researchers at Carnegie Mellon University is a great example of this. They have combined several LLMs for problem solving, code generation, and experimental design, with the ultimate goal of accelerating R&D in chemical research.
-
Fluidity of problem solving approaches: Librarian-like AI will vastly increase the visibility of alternative approaches and technologies to overcome technical hurdles, for instance, by finding solutions to similar problems in unrelated industries. This will have significant implications for project team composition and mentality. The application of generative AI approaches to well-established problem solving frameworks, such as TRIZ, could create significant productivity gains in this domain.
Across both dimensions, the design and interface of AI tools is vital if they are to achieve widespread user adoption, and thus deliver benefits. They have to be integrated within existing ways of working that staff are comfortable with and augment their capabilities. For instance, one of our clients (a large chemical player) has developed a transversal LLM team to support both “horizontal” and “vertical” initiatives across its vast R&D community.
Collaboration
The limitations of current collaborative data sharing and knowledge management systems have been amply described. Put simply, critical knowledge and data are often not accessed and used because they:
-
Are not properly codified/tagged
-
Sit in inaccessible locations
-
Exist in different file formats and/or different languages
-
Are “tacit” (i.e., part of people’s professional experience)
-
Are spread across multiple different ecosystem players
-
Are not being sought or shared by individuals for a variety of reasons
These obstacles are significant for deploying AI to help create perfect innovators because AI needs data by the truckload and people need new knowledge and insights to make new discoveries. Fortunately, AI and other digital tools are also part of the solution, for example:
-
Advanced knowledge search: LLMs can be combined with knowledge graphs and vector databases to create new solutions that combine natural language processing with content and contextual search. ADL has recently deployed this type of tool for its own internal use.
-
Intelligent triggers: AI tools can be applied to provide prompts to initiate the transfer of information or catalyze learning activities. Examples include predicting users’ future needs and prompting action, providing tailored knowledge debrief protocols at key milestones, and automating translation and tagging to generate quick reference lists for specific topics.
-
Knowledge management aids: AI can support documentation, search, transfer, and learning. For example, generative AI can suggest non-obvious and/or lateral information in searches and generate new content from unstructured data (such as text/video/audio) for knowledge capture. Graph data technology makes it simpler to identify previously hidden relationships within vast amounts of textual data within repositories.
-
User experience enhancement: New tools can be deployed to make interfaces easier to use than not to use. For example, adoption engineering can be employed to make sure knowledge management and learning solutions “go viral” and deliver behavioral change with seekers, while gamification encourages owners to share information. Within repositories, powerful data visualization interfaces make understanding and prioritizing information easier.
Ambidexterity
While simply applying AI technology and tools to an existing lab structure has already had benefits, it is unlikely to result in any major breakthroughs (or even smaller ones) unless necessary changes are also made to the wider organizational context and operating model. After all, why would AI-enabled breakthroughs sail by well-known obstacles that plague other types of breakthrough innovations, such as inept decision making and misaligned incentives?
The lab of the future needs to embrace ambidexterity, balancing optimal exploitation of today’s innovation space and exploration of tomorrow’s growth domains — indeed, this why the T-profile of knowledge integration and knowledge expansion is essential.
R&D organizations that are geared toward exploitation tend to apply standardized formal structures and processes, supported by a performance-driven culture with KPIs focused on efficiency, cost reduction, scale, and productivity.
Those that are focused on exploration tend to have more lateral and fluid structures to allow greater responsiveness (for example, based on communities of expertise or guilds). Processes follow agile thinking and focus on iteration, rapid validation of ideas/sparks, and more freedom to follow “big bet” projects. Steering and incentives are more rooted in purpose and direction setting than individual project control.
Most R&D organizations, in practice, lean toward one of these archetypes or the other. ADL’s “Ambidextrous Organization Assessment database” finds that only 15% of companies are fully ambidextrous, with the majority (55%) optimized for exploitation (scale and productivity). A large majority of industrial R&D organizations still need to achieve ambidexterity, yet without it, the full potential of AI is unlikely to be realized.
So, what are the key features of ambidexterity in practice? Apple provides some useful pointers.
Lessons learned from Apple in ambidexterity
Apple is a longstanding innovation leader. We recently benchmarked Apple’s R&D function through interviews and desk analysis, confirming the importance of ambidexterity to its success. Notable features of its practices include the following:
-
Ambidexterity in decision making and performance management: Decision making depends on the type of project: more belief-based for design initiatives versus hard data to drive technical hardware specs. The steering of individual projects is much more closely linked to the ambitions and expectations of leaders (see next point) than dedicated KPIs, with team reputation seen as a more effective “control mechanism” than fixed indicators. R&D employee incentives are usually based on overall company performance rather than individual projects/products.
-
Ambidexterity in people management: Leaders are required to balance deep expertise and immersion in details with willingness to collaborate, with staff continuously assessed on these criteria. Apple follows the “experts lead experts” rule, requiring leaders to constantly learn in new areas as they become relevant to their area of responsibility. Clear distinctions have been made between topics to own, delegate, learn, and teach.
-
Ambidexterity in structure: Despite its size, Apple is purposely following a functional, rather than project-based, organizational model. In combination with other practices, it uses an expertise-driven approach and reduces the number of executives involved in decision making. While it still adopts project-driven ways of working, rather than fully formalizing this through organizational structures, it places much emphasis on a few selected project leadership roles, which are taken very seriously.
Comparing Apple R&D to a typical industrial company shows major differences in the ambidexterity balance (see Figure 3).
Two findings stick out from the comparison. First, whereas Apple R&D has embedded both “explore” and “exploit” in all organizational dimensions, industrial companies tend to optimize for “exploit” following predictable and well-controlled practices. Second, many industrial R&D organizations have dedicated units, and sometimes locations, designed for breakthrough innovation (“explore”), but this does not seem to be Apple’s formula for breakthrough success, which instead resides more in its whole approach to steering and transformation and managing people and culture.
Finally, Apple also exhibits the other key characteristics of the people-centric lab of the future, which we have already discussed. AI democratization and leadership are fully embedded within its functional organization as key guiding principles:
-
Democratization: A distributed (democratized) approach to AI classifies it as a crucial skill and requires the broader leadership to reach critical skill levels. It has no separation between “innovating with AI” (for example, increasing R&D productivity) and “innovations with AI” (such as improving the Siri functionality of iPhones).
-
Collaboration: Apple embraces its knowledge ecosystem, with high permeability in terms of AI experts moving in and out of the company routinely. It relies heavily on a managed ecosystem of high tech and software companies, academia, and startups.
Insights for the Executive
The scope and potential of the AI-driven, people-centric lab of the future is enormous. Resources are always limited and often spread thinly, which makes prioritizing (what is most important) and phasing (what do we do first) according to what matters most for the company’s strategic objectives crucial.
The guiding principle is “build for advantage, buy for parity”: focus efforts on must-win innovation battles based on superior technical capabilities, while adopting proven best practices and commercially available solutions for less important topics. For example, a pharmaceutical company may decide to deploy AI mainly to supercharge its core R&D activity of new drug discovery, while a food ingredient company might focus on AI-enhanced digital services around personalized nutrition and formulation. The principle is the same, but the focus is different.
These strategic innovation priorities can then be mapped to the T-shape model presented in this article: what are the implications to AI-driven knowledge integration and expansion? Do not get bogged down in technologies at this stage, but instead concentrate on what would need to happen along these two dimensions to make your people into “perfect innovators.”
This will give you the solution promises of AI that are specific to your company, which, in turn, will define the blueprint for your people-centric lab of the future. Focus on developing the three key components:
1. Democratization
-
Identify and prioritize AI use cases and adoption, integrated across the R&D and innovation cycle, with defined AI skill-level targets and competences.
-
Focus on end-to-end processes so technical experts can seamlessly adopt AI into their working routines.
-
Ensure no separation between “innovating with AI” and “innovations with AI.”
2. Collaboration
-
Develop and build out future requirements in terms of data architecture and engineering, as well as user experience.
-
Strengthen and diversify the wider innovation ecosystem and increase permeability between roles, teams, and the company at large.
-
Redefine the skills and expertise that are needed and use this to drive hiring, training, and team composition.
3. Ambidexterity
-
Strengthen the role and caliber of project leadership and solution engineering and increase the iteration steps in end-to-end innovation with more frequent optimization.
-
Focus on optimized decision making — reduce hierarchies and redesign governance to differentiate between types of decisions.
-
Foster an innovation culture that is familiar and comfortable with uncertainty and ambiguity — essential for being able to experiment and learn along the AI adoption journey.
AI is often portrayed in the popular media as a threat to people, threatening to take over their jobs and make them surplus to requirements. While the far future is impossible to predict, in the coming years at least, AI is paradoxically one of the most powerful tools we have to put people back at the center of industrial enterprise, where they belong.
Notes
[1] In this article we will use the words “innovation” and “R&D” more or less interchangeably. With the former we imply a broader concept of which R&D can be considered an integral part.
[2] 9th Arthur D. Little Global Innovation Excellence Benchmark, June 2023 https://www.adlittle.com/uk-en/insights/report/good-great-enhancing-innovation-performance-through-effective-management-processes
[3] “The Laboratory of the Future,” Prism S1 2020 https://www.adlittle.com/uk-en/insights/prism/laboratory-future
[4] https://www.nature.com/articles/s41586-023-06792-0
[5] https://www.nature.com/articles/s41586-023-06924-6
[6] See “Be Careful Out There — Understanding the Risks of Deploying Artificial Intelligence” elsewhere in this issue.
[7] “Generative Artificial Intelligence: Toward a New Civilization?” Blue Shift Report, October 2023 https://www.adlittle.com/en/insights/report/generative-artificial-intelligence-toward-new-civilization
[8] “Taking Control of AI,” Prism S2 2023 https://www.adlittle.com/sites/default/files/prism/ADL_PRISM_S2_2023_Taking%20control%20of%20AI.pdf